A Novel Graph-Based Framework for Classifying Urban Functional Zones with Multisource Data and Human Mobility Patterns
November 6, 2023
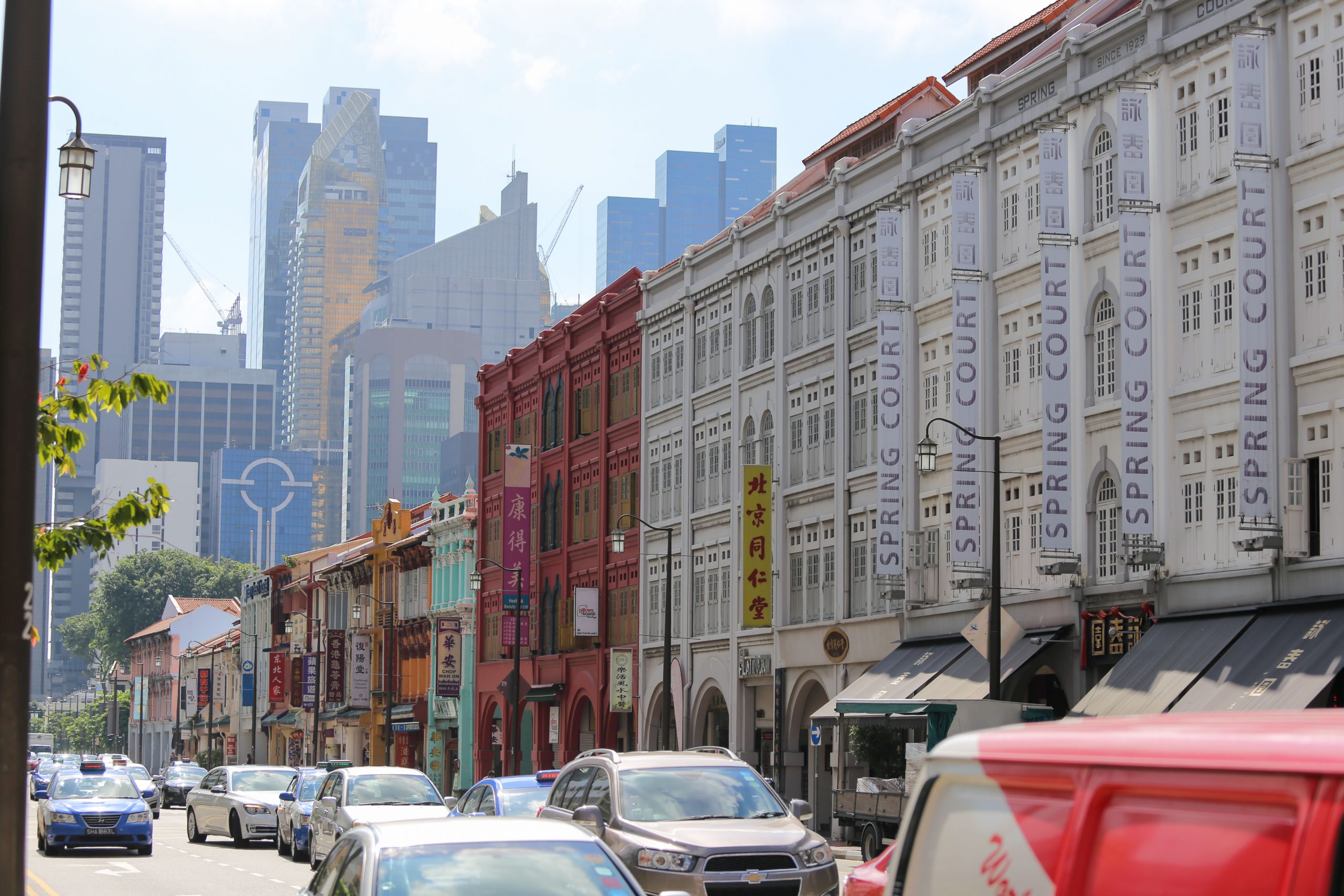
Since 1999, GIS Day has been held annually in mid-November to celebrate the technology of geographic information systems (GIS). GIS technologies capture data about spatial relationships across Earth’s surface and have important applications in urban planning and zone classification. However, specific applications such as classifying urban functional zones (UFZs) into industrial, residential, and other categories necessitate the use of different types of data on physical and social features of places to make accurate classifications. How should urban planners and researchers incorporate multisource geospatial data in a way that accounts for the dynamic ways people move across and use spaces in real life?
In ‘A Novel Graph-Based Framework for Classifying Urban Functional Zones with Multisource Data and Human Mobility Patterns’ (Remote Sensing, 2023), Ms Jifei Wang (NUS Geography and the Chinese University of Hong Kong Geography), Associate Professor Chen-Chieh Feng (NUS Geography), and Professor Zhou Guo (Sun Yat-sen University School of Geospatial Engineering and Science) propose a new graph-based framework to accurately classify UFZs at scale, leveraging multisource data and human mobility patterns. The generalisability of the framework was a key consideration of the researchers, therefore allowing their graph-based framework to be suitable for adaptation and application in other fields requiring heterogeneous data fusion.
Firstly, the researchers’ framework involves extracting high-level scene information through deep neural networks, leveraging High Scene Resolution (HSR) remote sensing imageries. Their AI model automatically learns high-level features of remote-sensing images, looking at scene (i.e. image) composition and its constitutive objects/buildings in 2D and 3D to hazard a guess at what UFZ it is. Subsequently, they also feed their AI model multisource semantic embeddings, which are constructed based on physical features and social sensing features. These semantic embeddings can include location-based social sensing data, such as points of interest, social media check-in data, mobile phone positioning data, and GPS trajectory data.
Secondly, their framework follows up by constructing a UFZ mobility graph, spatially joining trajectory information of human journeys to build topological (i.e. spatial) connections between different places. For their studies on Zhuhai and Singapore, the researchers used data on taxi travel information.
Lastly, their framework builds the final model by transforming UFZ segments and multisource semantic features into nodes and embeddings in the mobility graphs, such that the model can classify UFZ quickly and accurately. The researchers stack multiple layers of graph convolutional networks (GCNs) that capture information about larger neighbourhoods and spatial dependencies between UFZ. Finally, they use the Graph SAGE model to extrapolate the data it was trained on, in order to generate embeddings for previously unseen nodes by applying what it has learned on an aggregate scale. This enables estimation of the UFZ for new spaces outside the training dataset.
The researchers highlight that classifying UFZ accurately at scale is crucial for urban planning because UFZs are seen as the basic spatial units for studying the features of the urban environment. Hence, the researchers’ novel graph-based framework that uses the Graph SAGE model for high accuracy has the potential to streamline urban planning practices. This then allows planners to derive an ideal UFZ configuration to alleviate the negative consequences of urbanisation.
Read the article here.