FASS Global Research Forum: Day 3 Keynotes by Professor Anindya Ghose, Professor Zeng Yi, and Professor Munmun De Choudhury
September 24, 2021
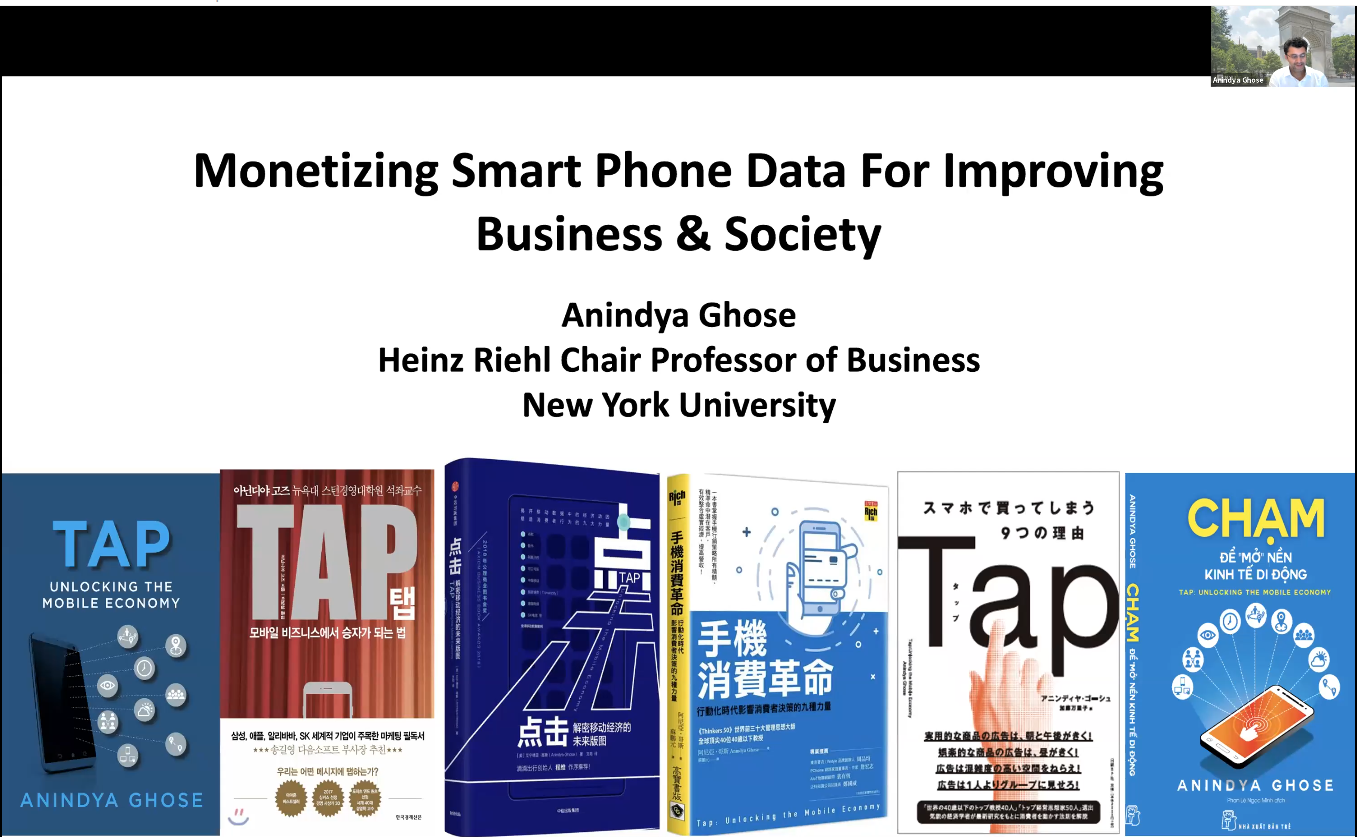
Professor Anindya Ghose (New York University) delivered the FASS Global Research Forum’s fifth keynote, ‘Monetizing Smart Phone Data for Improving Business and Society’. His research examines what high achieving companies will excel at in the near future, and he emphasized that these firms will need to successfully identify the unknown needs of their known and unknown customers. Firms must have a big presence in the mobile economy, which is a huge data source for customer behaviour. They must also invest heavily in analytics, which will provide a toolkit for computational social science research. Thirdly, they need to be able to distinguish genuine content from fraudulent content, which is possible with blockchain technology.
Prof Ghose discussed the nine forces that shape the three trillion dollar mobile economy, influencing consumer behaviour, often in combination: location, time, context, omni-channel, crowdedness, weather, trajectory, social dynamics, and saliency. He talked about his study on contextual targeting in Seoul, which was employed to boost online-offline (O2O) synergies. Data on commuters and urban areas were harnessed to enable business opportunities such as efficient food deliveries to commuters stuck in traffic. Prof Ghose touched on related studies, including one that looked at how billboard advertising could be coupled with mobile targeting (selecting users who had seen the billboard ad) to enhance O2O synergies, leading to a fourfold increase in voucher redemptions. He also explained trajectory-based targeting, which was found to more successful than other forms of targeting in studies inside shopping malls were consumers’ shop visit routes were tracked to gauge what they were looking for.
Prof Ghose next discussed how we can use smartphone data to improve our societies. He went through research he had carried out on using smart mHealth platforms to empower patients with diabetes and other chronic diseases, gauging the impact and sustainability of the technology on their healthcare outcomes. The study revealed that although mobile health applications were effective, personalized communication had a weaker effect than non-personalized communication. Prof Ghose stressed that in this context, consumers preferred companies to act more like butlers and less like stalkers. He added that his research is applying mobile data to related projects in public health as well as in education.

Professor Zeng Yi (Peking University and Duke University), together with Dr Wang Zhenglian (Duke University) and Associate Professor Feng Qiushi (NUS Sociology), delivered the FASS Global Research Forum’s sixth keynote, ‘Computational Social Science Innovative Methods/Software of Household and Living Arrangement Projections for Informed Decision-Making’. Dr Wang went over three types of methods and models for household projection or simulation: headship rate, micro simulation, and macro simulation. She stated that micro models can handle large state space with many covariates, retain relations of individuals, and provide rich output, making them powerful in detailed analyses of complex behavioural patterns and kinship simulations.
Macro models, on the other hand, are limited in the number of covariates and exclude complex relations among individuals, but are not limited to the sample size. They can comprehensively use census information as a starting point to project households of an entire population. Dr Wang pointed out that some macro household projection models require data on transition probabilities among different household types, which are not available from conventional data resources such as surveys, censuses, and vital statistics, and not closely linked to demographic rates. She explained that the ProFamy extended cohort-component model and method Prof Zeng developed requires only conventional data like demographic rates.
In ProFamy, the individual is the basic unit of analysis, rather than the household, and population/sex distributions and household distributions are forecast simultaneously. The model adopts an innovative computational strategy that does not require huge datasets. It calculates changes such as marriage, co-residence with parents or children, migration, and survival statuses. Dr Wang further explained that the model judiciously uses stochastic independence assumptions to face data reality. ProFamy uses the harmonic mean to ensure consistency between the sexes and between parents and children the projection model. It uses national model standard schedules and summary parameters at the sub-national level to specify projected demographic rates of sub-national regions. In contrast to the headship rate model, which cannot link to demographic events, the ProFamy model closely links projected households with demographic rates and summary measures on marriage, fertility, mortality etc.
Dr Wang and Assoc Prof Feng proceeded to explain what data is needed for the model and where to find it (noting the ProFamy is data-demanding), and how ProFamy has been applied in different projects and areas, particularly in the US and China. Assoc Prof Feng discussed research on how changes in population and household structure may affect future elderly disability in the USA, categorized by race and age, from 2000 to 2050. He also spoke about his project examining how changes in household structure may affect future housing demands in China’s Heibei province.
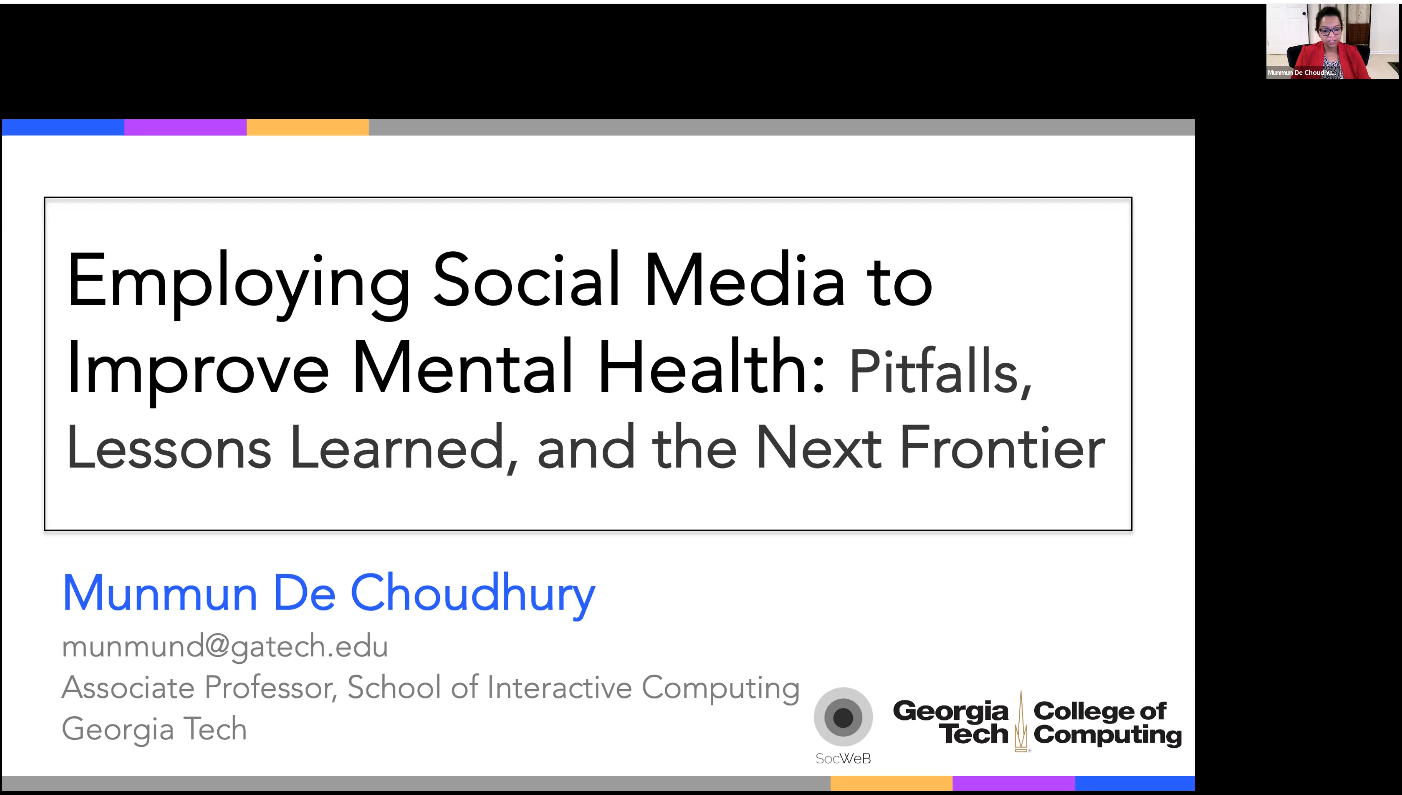
Professor Munmun De Choudhury (Georgia Institute of Technology) delivered the FASS Global Research Forum’s seventh keynote, ‘Employing Social Media to Improve Mental Health: Pitfalls, Lessons Learned, and the Next Frontier’. She explained that current crises are frequently accompanied by a variety of misinformation. Her research team studied how exposure to misinformation, as indicated by people’s sharing behaviours on social media (in this case, Twitter), affected their psychological wellbeing. They found that users’ anxiety levels increased approximately twofold. Moreover, people in vulnerable groups (such as lower socioeconomic status), experienced even higher levels of anxiety.
Prof De Choudhury also shared her study on how acute stress in college campuses increases following gun violence incidents. The team looked at 12 incidents of gun violence in 12 college campuses from 2012 to 2016, and a total of 113,337 social media posts on Reddit. The amplified levels of stress they found following the violent incidents deviated from the usual stress patterns on these campuses in that they were characterized by reduced cognition, higher self-preoccupation, and more death-related conversations. The violent incidents were found to have a profoundly negatively effect on those who were indirectly impacted, via social media.
Prof De Choudhury next discussed her research on how psychopathological symptoms worsened during the COVID-19 pandemic, but stabilized over time. They compared 16 million Twitter postings during the first two months of the pandemic with 16 million Twitter postings from March to May 2019. They found that the posts during the early days of the pandemic were indicative of a habitual settling into a ‘new normal’. In addition, people were using social media to seek social support for their stresses and combat the prevailing social stigma around mental illness. These findings can be used to improve policymaking around mental health issues and give insights into how to better support mental health during and after crises.
Prof De Choudhury then discussed her action research inspired framework that seeks to effect transformative social change and that incorporates computational social science research. She described how the framework was used in her project, ‘THRIVE: Designing and Deploying Clinical Tools Powered by Patients’ Social Media Data’. The study examined whether we can predict relapse using social media, focusing on schizophrenia patients’ social media data and how it revealed aberrations in their behavior. She also noted that their study’s model was evaluated for accuracy using clinical chart data on each patient that included an analysis of false negatives, or periods of relative health wrongly predicted as relapses. The study showed that the machine learning algorithm used in the model can be useful to clinicians as it provides personalized, context-sensitive data that may not be otherwise observable to them, which can benefit their patients.
Prof De Choudhury talked about a related project, ‘REDUCE: Realtime Ensemble Data for Understanding Suicide Epidemiology’, which investigates accurate forecasting of suicide rates across the US. The project developed a machine learning framework that allowed near real-time estimates of suicide fatalities and examined how the framework could be used to assist public health goals. The framework utilized multiple time series datasets collected and pre-processed from both social media and clinical sources to conduct time series forecasting for weekly suicide death counts that mimicked the natural data acquisition process. Using multiple data sources reduces bias towards single data sources, Prof De Choudhury noted. The second stage of the research features a deep neural network that is trained from the estimates of suicides from the first stage, and it succeeded in reduce the error rate in predictions to under 1 percent. This also demonstrated, as Prof De Choudhury pointed out, how important it was to combine traditional data sources with social media data sources to boost accuracy and counteract the problem of ‘big data hubris’ in computational social sciences.
Watch the video from Prof Ghose's 'Monetizing Smart Phone Data for Improving Business and Society' here.
Watch the video from Prof Zeng's 'Computational Social Science Innovative Methods/Software of Household and Living Arrangement Projections for Informed Decision-Making' here.
Watch the video from Prof De Choudhury's 'Employing Social Media to Improve Mental Health: Pitfalls, Lessons Learned, and the Next Frontier' here.